Room 141 and Teams: https://teams.microsoft.com/l/meetup-join/19%3ameeting_YzM0MjI2NTUtY2ZhYy00MWFlLWJkZTYtMDI3NTYzYzJkZWFj%40thread.v2/0?context=%7b%22Tid%22%3a%22be62a12b-2cad-49a1-a5fa-85f4f3156a7d%22%2c%22Oid%22%3a%22fbd28915-dda5-478f-8ecb-a3682dcf0c3a%22%7d
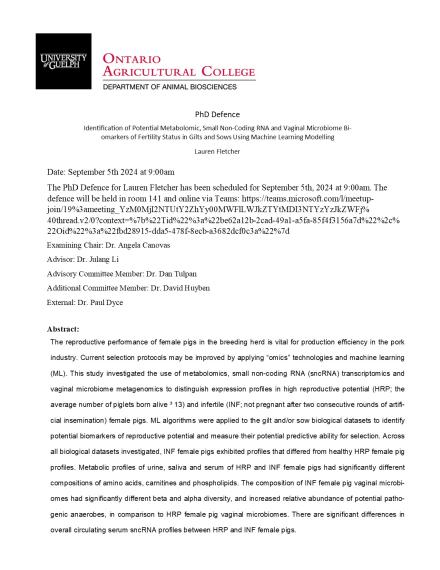
Identification of Potential Metabolomic, Small Non-Coding RNA and Vaginal Microbiome Bi-omarkers of Fertility Status in Gilts and Sows Using Machine Learning Modelling
The reproductive performance of female pigs in the breeding herd is vital for production efficiency in the pork industry. Current selection protocols may be improved by applying “omics” technologies and machine learning (ML). This study investigated the use of metabolomics, small non-coding RNA (sncRNA) transcriptomics and vaginal microbiome metagenomics to distinguish expression profiles in high reproductive potential (HRP; the average number of piglets born alive ³ 13) and infertile (INF; not pregnant after two consecutive rounds of artificial insemination) female pigs. ML algorithms were applied to the gilt and/or sow biological datasets to identify potential biomarkers of reproductive potential and measure their potential predictive ability for selection. Across all biological datasets investigated, INF female pigs exhibited profiles that differed from healthy HRP female pig profiles. Metabolic profiles of urine, saliva and serum of HRP and INF female pigs had significantly different compositions of amino acids, carnitines and phospholipids. The composition of INF female pig vaginal microbiomes had significantly different beta and alpha diversity, and increased relative abundance of potential pathogenic anaerobes, in comparison to HRP female pig vaginal microbiomes. There are significant differences in overall circulating serum sncRNA profiles between HRP and INF female pigs. The majority of differentially expressed miRNAs between HRP and INF female pigs have well-established roles in mammalian reproduction. ML modelling revealed predictive capacity of some of the investigated biological datasets for classification of gilt and/or sow reproductive potential. The vaginal microbiome and serum metabolome appear to have the most consistent patterns across gilt and sows, with the K-nearest neighbour algorithm performing moderately well in prediction of gilt and sow reproductive potential level using these biological datasets. A K-nearest neighbour algorithm using gilt vaginal microbiome data was best at predicting the reproductive potential level of gilts (F1 = 0.75, MCC = 0.89) and a Naïve Bayes algorithm using sow saliva was best at predicting the reproductive potential level of sows (F1 = 0.95, MCC = 0.89). Our studies suggest that ML algorithms and biological datasets could be used to improve overall production efficiency and profitability in the pork industry by improving upon current selection protocols.